How to analyze survey results and data: 6 easy methods to find answers
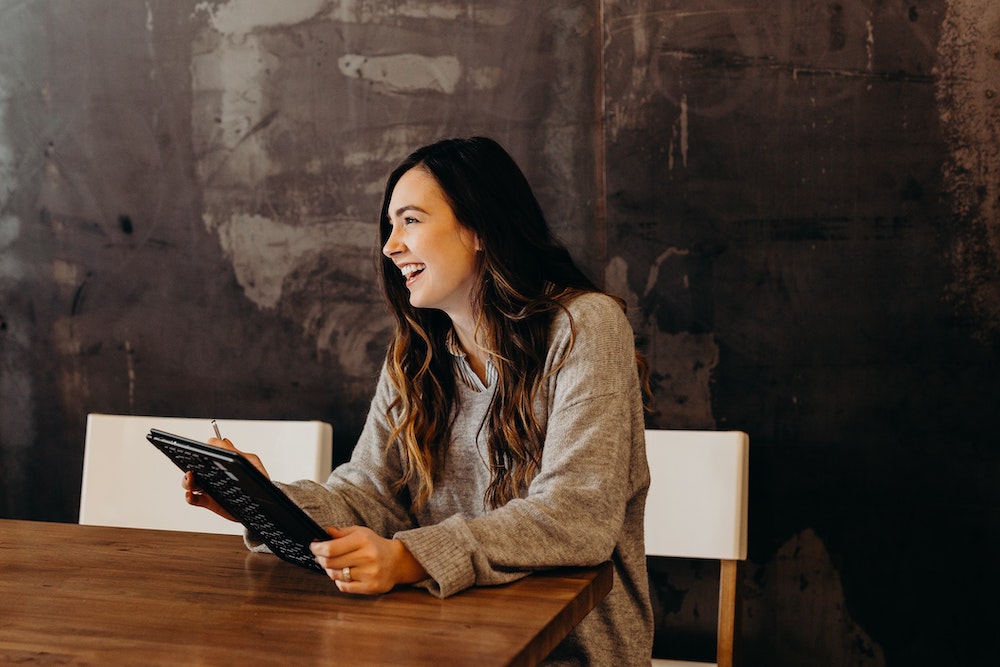
The most effective ways to analyze survey results aren't complicated. These methods will help you find answers & market insights from consumer survey data.
- What is survey data analysis?
- Where most survey analysis goes wrong (and how to get it right)
- Types of survey data you can analyze
- How to analyze survey your survey's results: steps and tips
- Essential questions to ask yourself
- Tips for improving survey data quality
- Survey analysis tools: finding the right fit for your needs
- FAQs about survey data analysis
Analyzing data from surveys is an underestimated skill. Those jazzy graphs and flashy dashboards from your survey tools are just the starting point. Without applying the right filters, asking critical questions, and challenging your assumptions, you could be looking at a completely different (read: incorrect) version of the truth.
You need to find unexpected connections, eliminate hidden biases, and approach your analysis with a healthy dose of skepticism. And of course, look at the results with your research goals in mind.
In this article, we’ll help you master the art of analyzing survey results, to go beyond the obvious. Well-informed business decisions start here!
Here are our top tips:
- Look at the results of your survey as a whole
- Take a look at the demographics of those who responded
- Compare responses to different questions to find deviations
- Find connections between specific data points with layered data
- Compare new data with past data
- Be critical, always
What is survey data analysis?
Survey data analysis is the process of examining, cleaning, transforming, and interpreting the information collected through surveys to uncover meaningful patterns and actionable insights. It involves converting raw responses into digestible information that can guide decisions for you, your team and your busiess.
Effective survey analysis isn’t just about tallying up responses—it’s about understanding the context, identifying trends, and connecting different data points to paint a complete picture of what your audience is telling you.
The goal is to transform seemingly disconnected answers into coherent insights that inform your strategy, whether that’s developing new products, refining your marketing approach, or improving customer experience.
When done right, survey data analysis bridges the gap between what your audience says and what your business does. It turns feedback into foresight, helping you anticipate needs rather than just react to them.
Where most survey analysis goes wrong (and how to get it right)
So what makes interpreting survey results so hard?
Most tools instantly showcase you the most interesting results, making you declare with confidence: “73% of our customers want feature X!” But do they really?
Survey analysis can go wrong in predictable and sometimes surprising ways:
- Focusing only on headline numbers: Yes, 73% might have selected that feature, but did you notice that only 12% ranked it as their top priority? Context matters.
- Ignoring segments and outliers: If you just look at averages, you’ll make an average marketing strategy that doesn’t activate your most valuable customers. Those averages look neat on paper, but they often mask crucial differences between customer segments. Your power users might have completely different needs than your casual browsers.
- Confusing correlation with causation: Just because two metrics move together doesn’t mean one causes the other. That apparent relationship between customer age and product satisfaction might be explained by a third factor you haven’t considered.
- Approaching data with pre-existing biases: When you’re convinced your new marketing campaign is brilliant, you’ll naturally gravitate toward the survey data that confirms your belief. Beware of cherry-picking!
- Not following up: running a survey just once is a common mistake. Follow up a few months to a year later to see if the decisions you’ve made have impacted the results.
The gist of our advice: don’t get carried away instantly. Use the filters and tools at your disposal. That way, you can look at results from different angles, which will help you turn your survey data from dry facts into a genuine competitive advantage.
Types of survey data you can analyze
Let’s start by categorizing the types of survey responses and data you’ll be looking at. Knowing what you’re dealing with will help you connect the dots, uncover patterns and extract actionable insights.
Demographic data
Digging into demographic helps you learn more about who is answering your survey, and how there are differences between certain demographic groups.
Certain education levels, ages, or even locations could have significantly different answers than the average, which is definitely something you should know.
Create your own segments as a way to cut your data based on your business’s very specific customer groups.
(p.s. you can do this really easily with Attest…)
Quantitative data
If you can count it, it is quantitative data. Think age, spending amounts, how often someone buys something or how they would rate the quality of a product.
Qualitative data
Qualitative data is harder to interpret, but incredibly important to give meaning to the numbers. It’s words, meanings, descriptions and feelings.
Want to dive a little deeper? Watch this to find out more about the differences between quantitative and qualitative research.
How to analyze survey your survey’s results: steps and tips
Time to learn how to analyze your survey results after you’ve sent out that beautiful survey and the data starts rolling in.
Before you get started…
Ask the right survey questions, find the right survey respondents, and choose the right tool.
We can’t ignore the importance of sending your survey the right way to the right people, because even the best analytical thinker can’t get any relevant customer insights if the research was sent to the wrong people and written in the wrong way.
Take the time to craft a survey that really gets to the heart of the research you’re running. While you’ll of course want your research process to be as efficient as possible, it really helps to get your colleagues’ views on your research questions. Don’t be afraid to tweak them to make sure your survey analysis will uncover the most valuable insights.
This ^ is what Attest’s Customer Research Team does day-in-day-out. They’re here to help brands create consumer and customer surveys that get to the heart of your research requirements.
For research findings you can trust, it’s also important to think about your sample size: how many respondents you’re sending your research to, and making sure they are representative of your target group. Make sure your sample size is big enough—which is easy to do with Attest, thanks to our pool of 150+ million high-quality respondents across 59 countries.
Consumer insights with expert support
Intuitive, easy-to-use tech combined with human research expertise at every step—that’s what you get with Attest. Start gathering quality insights today!
Book a demoNow let’s get into the specific steps to take when you start your survey analysis.
1. Look at the results of your survey as a whole
Before you analyze your survey responses, familiarize yourself with all the overall survey data, lay out your expectations and learn what is all in there, before getting too specific.
Look at the results and see what stands out to you, at first glance. What were you expecting to see or most curious about? It’s okay to have assumptions: simply make them clear to yourself before the survey is launched, and then see if they are debunked or confirmed.
You can also compare the results to similar surveys or studies to see if they’re in line with those findings.
Once you’re familiar with all that data, it’s time to zoom in on what results are most telling. The next few tips will help you find the key insights in your survey data.
We found out interesting extra details, like board game enthusiasts are much more likely to back something on Kickstarter and buy from certain small independent stores.
Becky McKinlay, Head of Marketing at Big Potato Games
It’s all in the details of your data. Find out how Big Potato Games won big with their survey analysis
2. Dig into the segments and demographics
What if you don’t look at the survey as a whole, but filter survey responses based on specific segments and demographic factors?
With Attest’s crosstabs and bespoke segments, for example, you can easily find interesting relationships between your most important groups and variables. You compare multiple sets of data within one chart to see if there are connections.
Play around with your survey data and see how specific it can get. For instance, women as a whole could be happy with your product, but when you zoom in on the younger generations, they might be driving down the average. That could be something to further focus on.
3. Compare responses to different questions to find deviations
It’s important to check for deviations before drawing conclusions, and possibly removing responses of people who don’t appear consistent in their answers.
For instance, someone might score you highly on product quality, but further down the survey they give a different opinion, in an open-ended question. When comparing data, try to identify patterns—and don’t just focus on the most positive answer for you.
4. Find connections between specific data points with layered data
There are various ways data can be connected, and understanding these types of connections will help you in your survey data analysis.
For instance, causation and correlation are two different ways data points can be connected, and they might change your views on the strategy that’s needed. It could also be the case that there is a confounding variable at play.
Here’s what that all means, if it’s been a while since you’ve opened a math textbook:
- Causation: when the value of one variable increases or decreases as a result of other variables changing, it is said there is causation
- Correlation: when one variable increases as the other increases the correlation is positive; when one decreases as the other increases it is negative.
- Confounding factor: A confounding variable is a third variable which influences both the independent and dependent variables.
5. Compare new data with you other data and insights
If you have any past data available, use it!
See how some things have changed and try to find explanations for them. Has customer satisfaction decreased drastically, but are you busier than ever? These could be related: for instance, you’re selling more, resulting in understaffing and longer waiting times.
Comparing your new raw data to past industry insights can also help you gather fresh ideas for the future. Take Bloom & Wild, who uncovered that red roses for Valentine’s are a thing of the past:
We found that 79% of people would prefer to receive a thoughtful gift rather than something traditional, like red roses. 58% of people thought red roses were a cliché.
And they actually came bottom as the least favorite gift that people had received for Valentine’s Day. So, that gave us confidence that we had correctly sensed growing reluctance towards those sort of Valentine’s Day clichés.
Charlotte Langley, Brand & Communications Director at Bloom & Wild
Read more about how Bloom & Wild followed up their hunch with research, and saw big results.
6. Be critical, always
Data analysis requires you to be skeptical. Be aware of how ‘true’ the data really is.
It can help to look into whether you have statistically significant research insights. A statistical significance test compares two groups and tells you whether a particular insight comparison is a result of chance or whether there’s more of a causal link.
We’ve built a feature in the Attest platform that tells you when you have insights that are statistically significant.
Essential questions to ask yourself
When you’re analyzing survey data, it’s easy to rush straight to the findings that excite you most. But seasoned researchers know there are fundamental questions you need to address before drawing any conclusions.
The following must-ask questions are adapted from Wake Forest University’s Institutional Research department’s guide on survey analysis (source):
“Did I get enough responses?”
More responses generally mean more reliable results, but it’s not that simple. Consider:
- Who didn’t respond? Non-response bias can skew your results if certain groups are less likely to participate.
- What’s my response rate? Always calculate and report this (responses divided by invitations sent). Even a seemingly low response rate (5-10%) can produce reliable estimates if your sample size is large enough.
- Do I have enough respondents per subgroup? If you plan to analyze differences between segments (like age groups or customer types), each segment needs sufficient representation.
“How confident should I be in these results?”
Before you proclaim that “62% of customers prefer our new design,” understand the statistical reliability of that number:
- What’s my confidence interval? This tells you the range within which the true value for the entire population likely falls. For example, with a 95% confidence level and a ±3% margin of error, that 62% might actually be between 59% and 65%.
- What’s my margin of error? This indicates how much your results might vary if you surveyed the entire population. The smaller your sample size, the larger your margin of error.
Always communicate these limitations when sharing your findings—it’s not just good practice, it’s ethical reporting.
“Are my responses representative of my target population?”
Your survey might have lots of responses, but if they’re all from one demographic when your customer base is diverse, you’ve got a problem:
- Compare respondent demographics to your overall population. If 70% of your customers are millennials but only 30% of survey respondents are, your results will likely be skewed.
- Consider weighting your data if certain groups are underrepresented. This adjusts the influence of each response to better reflect your actual population.
“Are these differences actually meaningful?”
Not every difference you spot in your data matters:
- Statistical significance tells you whether differences are likely real or just random noise. But remember: with large sample sizes, even tiny, practically meaningless differences can be statistically significant.
- Effect size measures how substantial a difference actually is. A statistically significant finding with a small effect size might not be worth acting on.
- Practical significance asks: “Is this difference big enough to care about?” A 2% improvement might be statistically significant but not worth a major investment to achieve.
“What’s the story behind the numbers?”
Quantitative data can tell you what’s happening, but qualitative responses often reveal why:
- Don’t skip open-ended responses just because they take longer to analyze. They often contain your most valuable insights.
- Look for patterns in the comments that might explain trends you see in your numerical data.
Tips for improving survey data quality
The quality of your analysis is only as good as the data you’re analyzing. Here are practical strategies to ensure your survey data is as reliable and valuable as possible:
Survey design improvements
- Keep it concise: Respondent fatigue leads to rushed, low-quality answers. Aim for surveys that take less than 10 minutes to complete.
- Use simple, clear language: Avoid jargon, double-barreled questions, and complex phrasing that could confuse respondents.
- Implement logic and skip patterns: Show respondents only the questions relevant to them based on their previous answers to improve completion rates and data quality.
- Include attention checks: Incorporate questions that verify respondents are paying attention, such as “Select ‘somewhat disagree’ for this question.”
- Balance your scale options: Provide an equal number of positive and negative options in rating scales to avoid bias.
During data collection
- Pilot test your survey: Run your survey with a small test group first to identify and fix any issues before full launch.
- Monitor responses in real-time: Check early responses for potential issues with question interpretation or technical problems.
- Set quotas for representative sampling: Ensure your sample matches your target demographic profile, implementing quotas if necessary.
- Offer appropriate incentives: Provide reasonable compensation that motivates thoughtful participation without attracting respondents who are only interested in rewards.
Data cleaning techniques
- Remove speeders: Flag and review responses from participants who completed the survey significantly faster than the average time.
- Address straight-lining: Identify and consider removing respondents who select the same answer option for all questions in a matrix.
- Check for logical inconsistencies: Look for contradictory answers across related questions that indicate the respondent wasn’t answering truthfully.
- Analyze open-text responses for quality: Flag responses containing gibberish, irrelevant content, or copied text.
Survey analysis tools: finding the right fit for your needs
Choosing the right survey analysis tool can make a world of difference in how efficiently and effectively you extract insights from your data. Here’s a guide to help you navigate the options:
Key factors to consider when selecting a survey analysis tool
- Ease of use vs. analytical power: Determine whether you need simple data visualization capabilities or advanced statistical analysis features.
- Integration capabilities: Consider how well the tool connects with your existing tech stack, from CRM systems to data warehouses.
- Visualization options: Look for tools that offer a variety of chart types and customization options to effectively communicate your findings.
- Collaboration features: If multiple team members will be working with the data, prioritize tools that allow for seamless sharing and collaborative analysis.
- Scalability: Ensure the tool can handle your current survey volume and grow with your research needs.
Draw meaningful conclusions from your survey data
When you want to start drawing conclusions, there are two important things to do: categorizing results, and visualizing them.
Categorizing will help you make clear distinctions between what is related and relevant, and what isn’t.
Visualization will help you better understand your survey data, but it also helps you communicate your survey data analysis in an effective way to stakeholders, marketing managers and anyone else who needs access to the insights.
When drawing up your marketing, product or sales strategy, these categories and visuals will help your strategic thinkers to test whether their ideas are in line with what the survey data is telling them.
Basically, for every decision they make, they have something to go back to and ask themselves: does this make sense based on the survey data analysis?
Presenting survey data and results
Time to organize your data and get it ready for your presentation. Here’s how you do that, in a way that will help everyone make the most out of your survey data analysis, without having to comb through a pile of data.
Choose which data to share (and which to keep to yourself!)
Make sure your survey report is complete, but also concise: leave out any data that didn’t turn out to be relevant. It’s important to keep it as a backup so people can refer back to it in case of confusion, but try to avoid stating the obvious.
Choose the right graph format
Bar, line or pie? If you want to help your colleagues interpret the results accurately, make sure the right format is picked. Here’s a little cheat sheet:
- Bar graphs: works for quantitative data, but can also show qualitative research data, for instance by counting sentiments.
- Line graphs: how quantified data evolves over time by tracking the ups and downs of the data.
- Pie charts: show the breakup of all the data into categories.
- Venn diagrams: overlapping circles show the logical relationships between two or more sets of items.
Creating an internal report
Simply sending out a spreadsheet with all the survey data won’t be the most time efficient way to deal with the data.
Take your time to craft a report that tells a story, to help people who need to make decisions based on the data understand the context of the survey.
Think about it this way: if someone gets hired, but wasn’t around when the survey was conducted, you’ll still want them to understand how the decisions were ultimately made. So, invest in creating a sleek report that covers the whole survey journey and most importantly: its results.
Make data more readable using infographics
Reading numbers can be exhausting and confusing. People have a short attention span as it is, and many people are better visual thinkers.
So if you want to make your report even better, shape your data into infographics that are easy to share and communicate.
This will help your creative colleagues’ brains and the results can then also be shared throughout the company, in a way which is engaging and understandable.
Use storytelling
Turning data into stories is certainly possible. For instance, you can take specific target demographics and make them more real by creating a detailed persona with a name and face to it.
Let them do the talking: write the survey responses out as if it were an interview, to help everyone better understand what is going through the customer’s mind.
Create a marketing strategy driven by data analysis
Knowing how to interpret and present survey results enables you to create a marketing strategy that is driven by accurate data directly from consumers. It doesn’t get any better than that.
If you need a tool that helps you create and analyze surveys in a powerful way, it’s about time you meet Attest.
When data starts coming in, you have everything you need in your dashboard to filter and analyze it.
And you don’t have to do it alone. If you want to make sure that you analyze survey data exactly right, find out how we can help you.
Consumer insights with expert support
Intuitive, easy-to-use tech combined with human research expertise at every step—that’s what you get with Attest. Start gathering quality insights today!
Book a demoFAQs about survey data analysis
Most survey tools come with reporting features and a dashboard that presents all the data, but it’s you who has to play with filters to find significant connections in the survey results. You can then create graphs that help you identify trends and track data.
It all starts before creating a survey: what is it you want to measure? Set a goal for your survey and build it based on that. You can analyze your survey results easily in your dashboard, playing around with filters to find connections.
With a lot of critical thinking, being wary of assumptions and keeping statistical significance in mind. For accurate survey data analysis, make sure you remove any data that’s wrong and incomplete before you start drawing conclusions. Plus, if possible, test the accuracy of the data with past or other relevant survey responses.
It all starts with formulating clear and concise research questions, and going from there. Select the right respondents and a tool that helps you analyze the results quickly and accurately.
Mixing and matching qualitative feedback with demographic data and numbers is tricky. Make sure you can lead open-ended responses back to specific groups of people and see how their answers match to other questions.
Tell us what you think of this article by leaving a comment on LinkedIn.
Or share it on: